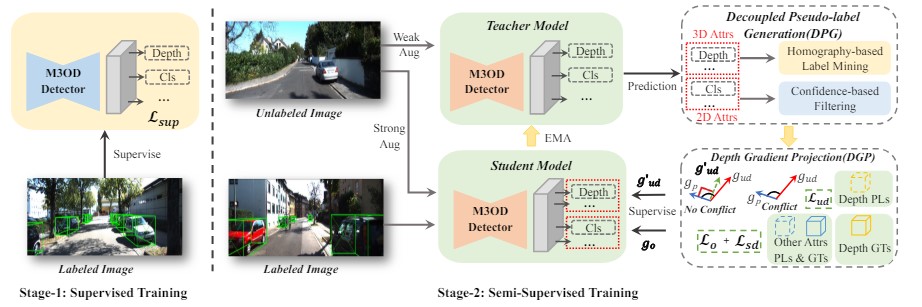
CVPR 2024
Decoupled Pseudo-labeling for Semi-Supervised Monocular 3D Object Detection
Jiacheng Zhang, Jiaming Li, Xiangru Lin, Wei Zhang, Xiao Tan, Junyu Han, Errui Ding, Jingdong Wang, Guanbin Li
CVPR 2024
中山大学人机物智能融合实验室
Human Cyber Physical Intelligence Integration Lab
- hcp@sysu.edu.cn
- 广州市广州大学城外环东路132号
Official Account
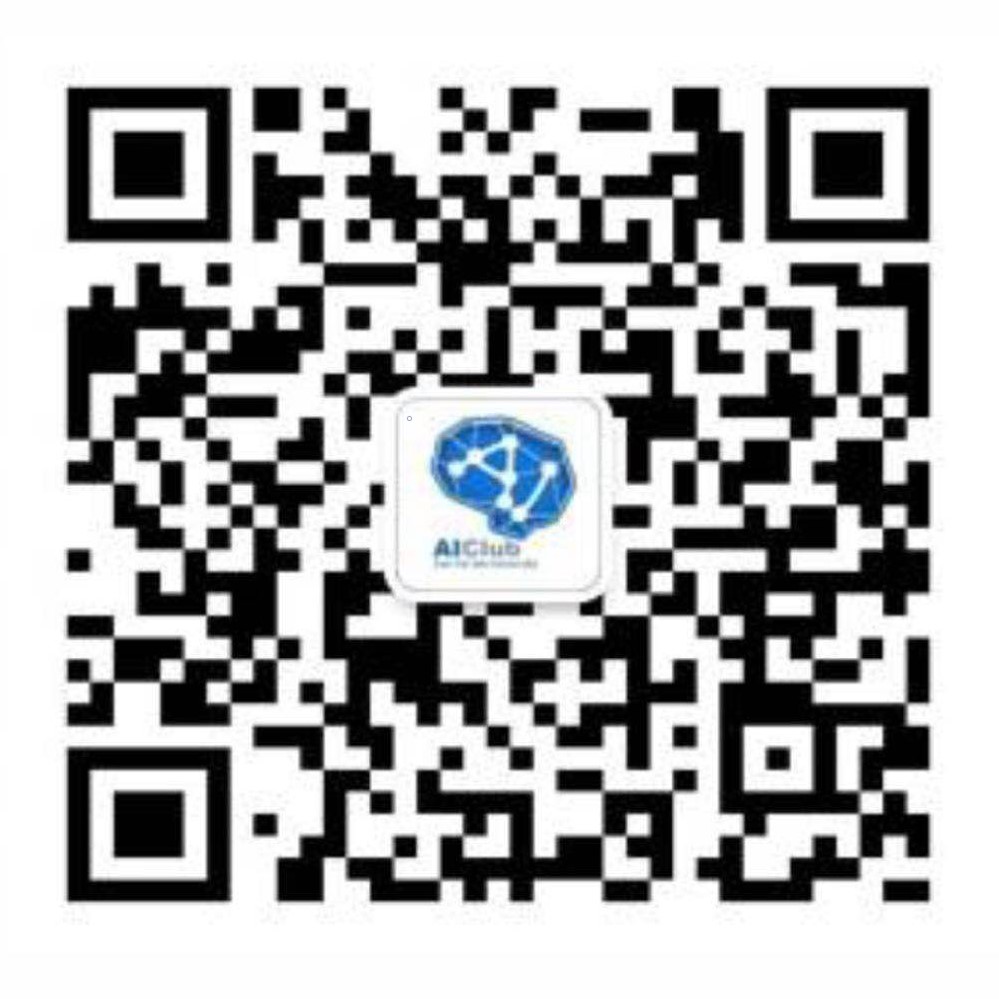
- Projects
- Computer Vision
- Multimodal
- Robotics
- Links
- Git-Lab
©2025 HCP in SYSU 广州慎思智能技术有限公司开发 粤ICP备2021037607号
©2025 HCP in SYSU 广州慎思智能技术有限公司开发
粤ICP备2021037607号